Last Updated on December 11, 2024 by Satyendra
Imagine your organization’s data landscape like a city, full of potential but difficult to navigate without direction. It is because of these complexities that a data governance framework is necessary.
It is not just about owning your data, it is about securing your organization’s ability to make better decisions, meet compliance requirements, and optimize the biggest asset of all, your people. But data governance always resembles one of those cliche business terms, everyone admits to its relevance while not everyone knows how to do it right.
In this blog, you will learn about the concept of a data governance framework, why it’s important for any given organization in the complex business world, and, most significantly, how you can implement an effective data governance framework that addresses your organization’s specific needs.
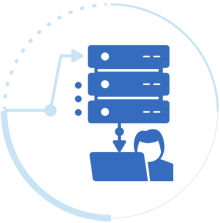
What is a Data Governance Framework?
In a nutshell, data governance is a formal structure used to own, manage, and use data in an organization. It serves as a reference guide that guarantees integrity, credibility, and availability of data to users who require the information.
But it’s not just a set of rules, it is a system of compliance measures so that everyone aligns with the rules on the books. An ideal structure defines responsibilities, measures, guidelines, and practices regarding the processes of collecting, storing, analyzing, and disclosing data.
Major Risk Management Components of a Data Governance Framework:
- Policies and Standards: The set of requirements that have to be complied with concerning data processing.
- Roles and Responsibilities: The question of who is responsible for data and its management.
- Data Quality Management: Using data that is accurate, complete, and reliable as a measure to achieve the organization’s goals and objectives.
- Technology Enablement: Governance-related tools and systems such as Metadata Management or Data Catalogs, etc.
- Monitoring and Metrics: Monitoring the outcomes of applying the framework on a second level of evaluation.
In simple terms, it ensures that all your staff members in your organization are on a common ‘data platform’ so that they do not create misunderstandings and avoid the misuse of data.
Why is a Data Governance Framework Important?
- Data Quality and Consistency– Data governance is not a database protection question but rather data utilization. Think about the opportunities that you can make efficient decisions with poor-quality information. Another key benefit of a strong framework is data quality, or at least the capability for any given team to trust the data to which it has access.
- Regulatory Compliance– The tough protection laws are being implemented in every country, be it GDPR or the HIPAA law. Applying a proper governance framework has a protective effect in the noncompliance field, avoiding great penalties and damaging the image of the organization.
- Security and Privacy– As cyber risk becomes more substantial, it is essential to protect critical data from such threats. Policies set up procedures that a firm needs to follow to avoid such acts of intrusion and violation.
- Operational Efficiency– When not well-governed, data is dispersed in an organization often resulting in redundancy and poor performance. These barriers are dismantled by a framework that makes the processes more flexible and easier to integrate.
- Data-Driven Decision-Making– If the data is well managed, then it becomes a powerful weapon and not a hindrance. Insights can be used with much more certainty, where data is accurate and manageable from the business perspective.
Data Governance Framework Models and Examples
No two organizations are similar to each other; therefore, there is no similarity when it comes to data governance. Here are some common models and examples to illustrate how frameworks can be tailored:
1. Centralized Framework
In this model, all governance activities are coordinated and performed by a single team or department. This approach is ideal for a small organization or one where there is no variation in data requirements.
Example: A regional hospital handling large numbers of patients’ records under a single compliance team that ensures the implementation of tight healthcare rules.
2. Decentralized Framework
In this context, governance duties are divided amongst various departments or groups. While this is flexible, it can only work if there is very good communication to ensure that the flow doesn’t contradict itself.
Example: A company with operations in multiple countries where marketers, financiers, and logistic personnel manage their data individually but under certain set standards.
3. Federated Framework
In this hybrid model, the major activities are coordinated and managed centrally, but the implementation is done at the decentralized level to ensure both standardization and flexibility
Example: An international banking and investment company where the main office decides on the rules at the same time where local subtleties of data handling are addressed.
Several established frameworks have set benchmarks for implementing effective data governance. Below are six notable frameworks, each offering unique methodologies and principles:
- DGI (Data Governance Institute)
- DAMA DMBOK
- McKinsey
- Eckerson
- PwC
- Deloitte
1. DGI (Data Governance Institute)– The framework comprises ten global aspects fundamental to data governance, captured by the acronym DGI: the reason, the object, the subject, and the manner.
Key Features:
- Goals, Metrics, and Funding: Key to business strategy since it facilitates revenue growth, efficient cost control, and organizational flexibility.
- Controls: Applies risk control measures in the governance structure and accomplishes the correction of the risks when necessary.
- Data Governance Office (DGO): Supervises the whole program, and works closely with partners to ensure compliance with policy and standards.
DGI emphasizes simplicity by categorizing its components into three core areas: rules, people, and processes.
2. DAMA DMBOK– The DAMA DMBOK concept map paints a wheel of data management with its hub as governance and the rim as nine data management knowledge areas.
Key Knowledge Areas:
- Data Architecture Management: Defines the organization’s data architecture and the relationships that exist among all data elements.
- Data Development: Emphasizes data warehousing structuring or architecture, construction, and updating.
- Metadata Management: It guarantees that data with high quality is captured, classified according to its type, and preserved.
3. McKinsey– In analyzing McKinsey’s framework, it is clear that the theme of organizational design is an essential prerequisite to data governance.
Core Components:
- Data Management Office (DMO): Establishes directives for actions, educates management, and makes certain that good government permeates all spheres of an organization.
- Domain-Based Roles: Supervise the day-to-day implementation of governance activities.
- Data Council: Responsible for the overall leadership of a strategic plan, managing issues that hinder execution, and enterprise coherence across domains.
McKinsey’s approach guarantees that governance will be aligned effectively in executing operations.
4. Eckerson– Eckerson Group has a six-layered approach comprising 39 components that happen to emphasize the people’s side of governance.
Key Layers:
- Goals and Standards: Explain precisely what governance programs are, and what their goals and approaches are.
- Processes: Tracking must show that efforts made align with goals: data quality and metadata cataloging, for example.
- Culture: Enables teamwork, and distribution of data and fosters organizational transparency.
One particular aspect of this framework is that it defines data governance and management roles as people, such as data owners and stewards or data curators.
5. PwC– This framework relies on existing frameworks: DAMA DMBOK and DGI; however, it has to consider the contemporary data environment.
Core Components:
- Governance Strategy: Stands as the starting point of data governance.
- Lifecycle Management: It guarantees that all policies address all the phases of data management.
- Stewardship and Enablers: Organized around ideas of people, processes, and technologies of governance.
Since the concept developed in this model embraces as extensive a view as possible of the data environment, it can easily accommodate shifts in that environment.
6. Deloitte– Deloitte’s framework deals with data value generation while keeping the risks in mind.
Key Elements:
- Policies and Principles: Acts as a reference to guide governance and management practices.
- Roles and Responsibilities: Describe what it means to have accountability structures.
- Processes: Describe the process of Populating, Editing & Updating the data set.
- Tools and Technology: Serve governance with solid tools and structures.
- Governance Controls: Benchmark governance initiatives, policies, Objectives and strategies.
Deloitte also emphasizes sustaining such tasks to deal with continuously emerging data to cater to challenges.
These frameworks, though diverse, share a common goal: reinventing information as a competitive weapon. Therefore, it is up to a given organization to decide on which model will suit their needs, size, and type of data best.
How to Create an Enterprise Data Governance Framework
The idea of building a data governance framework from the ground could be challenging as such but when divided into phases, it becomes much easier.
1. Define Clear Objectives
First and foremost, you have to determine the ‘why’ of your framework to design and implement it. Do you want to enhance the efficiency of data collection? Enhance compliance? Improve the quality of decisions that are made in the organization. Your objectives will determine how the framework is going to be developed.
2. Assemble the Right Team
A governance framework is not a one-man show. Construct a multi-competency group of IT specialists, analysts, lawyers, and company executives.
Assign specific roles, such as:
- Data Owners: Responsible for specific subsets of data, it does.
- Data Stewards: Is accountable for data accuracy and conformity.
- Data Users: Retrieve usable information for the view of analysis and decision-making.
3. Develop Policies and Standards
Establish rules for:
- Data classification (e.g., sensitive, public, confidential).
- Access controls (who can access what data).
- Data lifecycle management (how data is archived or deleted).
4. Implement Supporting Technology
Choose tools that support governance activities, such as:
- Data catalogs to make datasets searchable
- Metadata management tools to track data lineage.
- Data quality tools to automate consistency checks.
5. Document Processes
Create clear workflows for how data is collected, stored, accessed, and retired. Ensure these processes align with organizational goals and regulatory requirements.
6. Monitor and Improve Continuously
Governance isn’t static. Use metrics like data accuracy rates, compliance audit results, and user feedback to assess your framework’s effectiveness and make improvements.
Frequently Asked Questions (FAQs)
Q. Who Should Own the Data Governance Framework?
A. Ownership is contingent based on the organization’s structure. A Chief Data Officer (CDO) or data governance committee usually leads it, and it is done department-wise.
Q. What Are the Common Pitfalls of Data Governance?
- Lack of Buy-In: From the viewpoint of employees, governance can be seen as being a conformity mechanism unless the positive impacts are explained.
- Over-Complexity: There is some truth in the suggestion that using a framework with too many rules can slow you down.
- Neglecting Culture: Innovative governance frameworks must ensure that organizations engender a culture of data-oriented understanding among people.
Q. How Long Does It Take to Implement a Framework?
A. Building a foundational framework can take 6-12 months, but ongoing optimization is part of the journey.
Q. Do Small Businesses Need Data Governance?
A. Absolutely. Structured data management is valuable in even small organizations, particularly as they grow in size.
How Lepide Can Help
Lepide Data Security Platform is a powerful solution that can help organizations streamline their data governance processes. It automates tasks like data discovery, classification, and access control, ensuring compliance with data regulations. By monitoring user activity and generating insightful reports, Lepide helps organizations protect sensitive data, mitigate risks, and make informed decisions.
Conclusion
Creating a set of data governance guidelines is not only about completing a checklist, it should be about creating a sustainable strategy. Regardless of whether you are focused on compliance, better decision-making, or innovation, the correct framework turns your data from being a problem to an advantage.
Without governance, your data is like a party without a playlist, chaotic, overwhelming, and hard to enjoy.
Invest time into constructing a structure that will offer your business success in the future. In the current generation with increased use of technology and the internet, it is not only recommended but mandatory.
If you want to take a look on how Lepide can help in data governance, feel free to schedule a demo with one of our engineers today!