Generative AI is a game changer, reimagining the future of creativity, automation, and even cybersecurity. Models like GPT-4 and DALL-E, which can produce human-like writing as well as attractive images and software code, have opened up new possibilities for both corporations and individuals. However, with such great power comes significant hazards. Cybersecurity specialists are increasingly interested in generative AI, not just for its advances, but also for the vulnerabilities it creates.
In this article, we will look at the complexities of generative AI, including its functionality, security threats, and how businesses can effectively minimize these risks.
What Is Generative AI?
Generative AI is a branch of artificial intelligence that creates and produces new content, which can include text and image, audio and video, and even code. Unlike traditional models that classify or analyze information, these models generate completely novel, heretofore unknown outputs based on large sets of data with which they have been trained.
Generative AI systems are a part of deep learning and are mainly based on Large Language Models (LLMs) and neural networks. Some of them are programmed to analyze data and produce articles from the pattern they are given.
Real-World Generative AI Models
- GPT-4 (Generative Pretrained Transformer): Known for producing remarkably human-like text.
- DALL-E: A model capable of generating detailed images from text descriptions.
- MidJourney: Popular for generating artistic visual content.
The application of generative AI is significant in media, design, health, and many other industries. It includes gadget manufacturing, automated content creation, synthetic data generation, AI-assisted software development, and more.
However, as the advancement of technology continues to be realized, so does the abuse of technology which gives rise to key security and ethical questions.
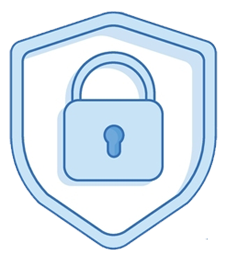
How Does It Work?
Generative AI has its roots in millions of data sets as well as highly elaborated algorithms. There are numerous text and image generation models, however the largest of them are transformer models, and many texts and images are sequential and it can predict based on the generated data.
The Building Blocks of Generative AI:
- Large Language Models (LLMs): These models like GPT-4 are trained on multiple datasets with billions of parameters they are so large and complex. In a wider sense, the scale of training enables these models to capture context, grammar, and syntax to a great extent. For instance, GPT-4 has a parameter of 175 billion that make it produce human-like language outputs.
- Neural Networks: Similarly to how the human brain works, all these networks are designed to learn patterns and relationship in the data. The) Taking advantage of training and reinforcement, the generative AI can be made to come up with new and unique outputs.
- Reinforcement Learning and Fine-Tuning: After training, there is fine-tuning with distinct sets of data which makes it hypertrophy-specific enabling organizations to develop an AI system that works like the one needed in a certain industry.
These are remarkable models but the scale that they hold comes with vast security risks.
Security Risks of Generative AI
Generative AI is one of the biggest opportunities we have but it also brings cybersecurity threats. Starting with the data leak and including AI voices or deep fake as a communication technique, this technology is rather dangerous for the interested business and government parties. Below are the major risks that generative AI poses:
- Frameworks of Data Leakage and Privacy Violations
The most serious issue with generative AI is that of data leakage. This is because generative AI models are designed on extremely large datasets, in some cases, they may even reproduce information which has been deemed as sensitive in the data on which models were trained. This can result into explicit incidences of invasion of the getUsers privacy..
For instance, OpenAI stated that at most 1-2% of the input that large language models utilize may be inadvertently exposed during the generative process, such as identifying data.
This problem becomes acutely sensitive in businesses with strongly defined regulation obligations, for example, the healthcare or financial services industries where a data leak may cause huge financial or reputational losses.
- Malicious Code Generation
Cybercriminals can also leverage generative AI to create malicious text, which can include malware and ransomware scripts. Burglars have already used GPT in generating complex phishing emails and even creation of code and it reduced the skill level a hacker needed for an attack.
A CheckPoint report shows that APT groups are now starting to use AI-generated phishing scripts in order to bypass standard security tools.
- Model Inversion Attacks
In the case of model inversion attacks, it is possible for the attackers to make a model and recover information from the training data set. This means explicit data could be at risk, let alone anonymized data, which in the hands of a cybercriminal would mean access to proprietary algorithms or personal data.
For instance, researchers at Securiti showed how the attackers can obtain private information through generative AI models, lacking appropriate security measures.
- Deepfake Creation and Fraud
Deepfakes that are created using artificial intelligence are only going to get better. It may be used for impersonation, spam, spreading fake news and for social engineering attacks. AI-powered voice cloning, for instance, makes it possible for hackers to pretend to be an executive or a famous personality, thereby making monetary losses to organizations and individuals.
According to a PWC study, deepfakes could cost up to $250 million in damage each year by 2026 because of fraud and misinformation.
- Bias and Ethical Issues
Mainly, the Generative AI models will work on pre-existing data and thus reinforce existing prejudices and archetypes. The problem with these models is that they engrained with such datasets and hence, will spew out results which will be unfair/discriminatory.
At the company level it can result in brand risk, legal lawsuits, and regulatory problems as fairness and ethicality are a major consideration in certain sectors.
How to Mitigate Generative AI Security Risks
Due to the current and future AI security challenges, it is crucial to adopt a holistic approach to overcome challenges of generative AI. Below are some key approaches:
- Data Privacy and Differential Privacy
The best way to reduce leakage of data is to clean training sets before using the data by stripping all identifying characteristics. These technologies must also be used to enhance differential privacy methods and ensure the models shield individual records such that sensitive data cannot be learned by attackers during the generative phase.
To protect data, big brands like Google and Apple have adopted differential privacy for their large scale AI models.
- AI Auditing and Monitoring
Periodic reviews of the AI models and constant observation of their outputs are necessary to identify any aggressive actions or security threats. The security risks arise out of the use of artificial intelligence and it is, therefore, crucial for organizations to set up a system that govern use of artificial intelligence to promote the right use of artificial intelligence systems.
For instance, PWC suggests third-party AI audit to be incorporated into particular organization to facilitate the adherence to the privacy and security laws.
- Encryption and Access Control
Dec 8 2021 Ina explanation for this revelation is the consideration of the fact that it is necessary to limit access to generative AI models. RBAC should be used by organizations as a way of allowing only the certified users to engage with the AI systems. Also, outputs of AI or training data that is sent or received have to be encrypted to avoid interception or alteration by some bad people.
- Human-in-the-Loop Systems
Using human supervision at strategized sections of the application of AI will be useful in detecting and preventing resulting inapplicable, bigoted or destructive outcomes. This human-in-the-loop approach ensures that AI systems remain accountable and ethical in their operation.
Generative AI Security with Lepide
In addressing the security challenges of generative AI, Lepide Data Security Platform offers essential solutions to mitigate associated risks effectively. With comprehensive monitoring of data interactions, permissions, and access, Lepide enables organizations to detect and respond to suspicious activities and potential security breaches in real-time. This is critical when managing sensitive data in generative AI environments, where the risk of unauthorized access and data exposure can be high.
Additionally, Lepide provides detailed audit trails and reporting capabilities that help organizations maintain compliance with data privacy and security standards, reducing the risk of regulatory violations. By leveraging advanced AI-driven insights, Lepide also offers anomaly detection to identify unusual patterns in data usage and access, alerting teams to potential threats before they escalate.
Through automated reporting and customizable alerts, Lepide helps organizations proactively secure their data and uphold compliance, ensuring that they are prepared to meet the unique security challenges posed by generative AI technologies
Conclusion
Generative AI is reshaping the future of technology, but its security risks demand serious attention. From data leakage to AI-generated malware, these threats are real and evolving. However, the solution is not to avoid AI but to secure its use through proactive measures such as encryption, monitoring, and ethical governance.
By combining strong security practices with human oversight, organizations can safely unlock the potential of generative AI. The key lies in balancing innovation with responsibility, ensuring that AI is both a tool for progress and a model for secure, ethical technology in the future.
If you want to know more about how Lepide can help, feel free to schedule a demo with one of our engineers today.