Last Updated on January 23, 2025 by Deepanshu Sharma
What is Data Governance?
Data governance can be defined as the overall management of data, including its accessibility, usability, consistency, and protection in any organization. It refers to the practices that concern the acquisition, holding, utilization, and disposition of data. The main goal is to ensure that data is up-to-date, integrated, and shared only by the selected individuals who have the right to see it, but it cannot be used by other users.
What is the Importance of Data Governance?
In the absence of proper data governance, achieving compliance and security can feel like navigating the ocean without a compass. The importance of data governance can be distilled into several key points:
- Regulatory Compliance: Due to the enactment of the GDPR and CCPA, among other laws such as the Data Protection Act, the use and handling of data has become the legal responsibility of organizations. Non-compliance leads to fines and damage to the company’s image.
- Data Quality Assurance: Data quality is the foundation of any kind of analytics and business intelligence. Data governance has been defined as the process through which data can be rendered fit for consumption in organizational decision-making processes because positive attributes such as accuracy, completeness, and reliability are accorded to the data.
- Operational Efficiency: Efficiency gains are realized through streamlined data management leading to the removal of many costly duplicated work and variations.
- Risk Management: Adhering to data governance policies also leads to risk management concerning data breaches, fraud, cyber threats, etc.
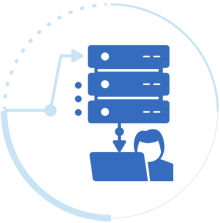
Benefits of Data Governance
Data governance benefits are numerous, extending well beyond regulatory compliance and are delivered by a good data governance strategy. Some of the key benefits are:
- Enhanced Data Quality: Data governance ensures that all the data incoming to the organization and existing within the organization is fit in terms of quality, reliability, and utility. This in turn results to accurate analysis and information sharing.
- Consistency Across Departments: Every large organization faces the problem of having data silos in which departments rely on data that is not standardized. In turn, governance connects these silos and standardizes the working with the data.
- Better Customer Trust: Transparency about data collection, storage, and usage is beneficial in establishing trust with the customer. Data governance is, therefore, a good sign that a given company is willing to uphold the right standards of data practice.
- Cost Savings: Eliminating redundancy, mistakes, and the need for shutdowns saves an immense money. Even data processes are less resource-consuming by automation of various processes.
- Competitive Advantage: With a strong data governance structure in place, organizations can utilize data and gain insights to foster an agile environment that innovates and sets new standards for competitors.
How to Implement a Data Governance Strategy
- Establish Goals and Objectives: It is critical to declare success or the state that your data governance should achieve before beginning the process. Do you seek to enhance compliance? Increase data quality? Improve decision-making? Mastery of clear objectives is at the root of the exercise.
- Form a Data Governance Committee: Establish a multi-disciplinary team that includes members from IT, legal, compliance, and other business lines. The tasks that entail the development and implementation of policies and procedures will be handled by this team.
- Define Data Policies and Standards: Ensure that detailed policies on user access to information, utilization, archiving, and protection are documented appropriately. For example, about data access identify who is authorized to work with the customer-sensitive information and under what conditions.
- Implement Technology Solutions: Make use of data governance software such as data cataloging tools and data lineage trackers as wll as quality assurance software systems in enforcing the rules of governance and monitoring status.
- Assign Roles and Responsibilities: Appoint people with specific responsibilities over data in the sense that data quality is maintained and data security assured. It is vital to write down every role on the list and establish precisely what responsibilities each person is to bear.
- Train Your Workforce: Inform employees on the need to adhere to data governance as well as what is expected of them in regard to compliance and quality. Training is useful to make sure that every employee on the premises understands governance objectives.
- Monitor and Adapt: Periodically check up your data governance policies to be sure you are still using the best one. Some examples of such metrics include data quality scores, outcomes of compliance audits, and risk assessment scores.
Common Challenges in Data Governance
While the benefits are substantial, implementing data governance is not without challenges:
- Cultural Resistance: The workforce is unlikely to adapt fast to changes and they regard data governance as an emerging task.
- Data Silos: Integrated governance refers to the alignment of different systems, and disparate data systems can therefore hamper the implementation of an integrated system.
- Resource Constraints: Insufficient staffing, both in terms of staffing people and technology constrains the advancement.
- Complex Regulatory Landscape: Studying multiple regulations of the international and industry level is challenging.
- Data Volume and Variety: The number of data categories and their variety may cause difficulties in governance initiatives.
Data Governance Roles and Responsibilities
Effective data governance necessitates clearly defined roles:
- Data Owners: Professionals managing specific types of data that need to have accurate and protected.
- Data Stewards: Data guardians, who operate at a regular employee level and ensure the usage of governance policies and standards.
- Data Custodians: IT personnel who are tasked with handling data most securely and transporting it safely.
- Data Users: The number of data categories and their variety may cause difficulties in governance initiatives.
- Data Governance Council: An organization’s senior management that supervises and leads the process of data governance and handles complex vital problems.
Key Pillars of Data Governance
Data governance cannot be achieved without emphasis on the following key aspects. All these pillars are crucial in achieving the goal of integrity, confidentiality, and accessibility of data across the organization. Let’s delve deeper into these pillars:
1. Data Quality Management: Data quality is the key concern for data governance. When data quality is low, decisions are wrong, processes are slow and resources are wasted. This pillar focuses on ensuring that data is
- Accurate: Completely clear of mistakes or inconsistencies.
- Complete: All the obligatory fields and records are completed and accessible.
- Consistent: There are no value dilemmas between datasets.
- Timely: They reflect current data that are relevant to their intended purpose.
In high-quality data, organizations use activities such as validation rules, cleansing processes, as well as monitoring systems. For instance, some features may alert readers to consider inconsistencies in financial data or lack of certain customer details.
2. Data Security and Privacy: As cyber threats rise, and regulatory requirements become more strict, data protection is a must. This pillar provides security to the information from access, incoming threats, and misuseKey components include:
- Access Controls: Specify who has the right to view data and under which conditions.
- Encryption: Encrypt data and its transmission to and from its destinations as well as data stored on devices and databases.
- Incident Response Plans: Ensure that they have measures in place to respond to security risks as quickly as possible.
- Privacy Compliance: Follow laws such as; GDPR and CCPA to ensure that individual’s data rights are observed.
For instance, a healthcare organization needs to safeguard patients’ records and follow HIPAA rules to ensure that their medical history and cost details are not exposed to anyone.
3. Data Architecture: Data architecture is the structural framework used in defining the ways of collecting, storing, integrating, and sharing data in an organization. Any properly designed data architecture must allow for scaling and optimize the data transfer.
Key aspects include:
-
- Centralized vs. Decentralized Models: Depending on the requirements, it is possible to have a centralized data store as well as have individual divisions or departments for storage.
- Data Integration: Integrating information from different information systems such as the CRM and ERP systems.
- Cloud vs. On-Premises: Determine which type of storage is best for data: its cost, usability, and protection level.
A retail company, for instance, may have a centralized data warehouse to consolidate sales data from physical and online stores and update inventory accurately in real-time.
4. Metadata Management: Metadata is sometimes referred to as “data about data.” It makes quantitative data meaningful and manageable by making it more comprehensive, retrievable,, and useful.
Key elements of metadata management include:
-
- Descriptive Metadata: Tells the content of data or the title of the document, when it is created, etc.
- Structural Metadata: Describes the structure by use of tables and columns which define how the data is arranged.
- Administrative Metadata: Contains the data on permissions, ownership, and revision history.
Managing metadata effectively helps enhance the way data can be found and used within an organization. For example, adding more metadata to a data catalog helps users to search for datasets, without having to call IT support.
5. Data governance policies and standards: Policies and standards are the regulatory guidelines that anyone dealing with data is bound to follow. The controls provide a proper method of handling data and are useful in meeting organizational or external regulations.
Key policies include:
- Data Retention Policies: Determine what is the retention period of data before archiving or deleting such data.
- Data Classification Standards: Subcategorize data according to its relevance and level of sensitivity (e.g., classified, open).
- Usage Guidelines: People must know how data can be used and get to it or exchanged among themselves and other members of the community.
All stakeholders must be informed of these policies and they should be updated from time to time.
6. Data Stewardship
Data stewardship is all about the delegation of responsibility regarding data assets. The last For example, of assigning leaders for datasets and their responsibility to enforce compliance with governance rules.
Responsibilities of data stewards include:
- The problem of maintaining the data accuracy and completeness is also addressed.
- Clearing up issues relating to conflicts or contradictions.
- Serves as an intermediary between technical support and commercial clients.
In an organization, a finance department may employ a data steward responsible for ensuring that the department’s data conforms to set accounting standards and audits.
7. Compliance and Risk Management
This pillar centers on the need to ensure data governance fits the legal requirements in use as well as drawing way to risks related to misuse or loss of data.
Key activities include:
- Audits and Assessments: Perform periodic checks so that compliance issues that may plague a certain function can be readily pointed out.
- Risk Analysis: Assess possible threats including compromised data, legal repercussions, and countermeasures.
- Regulatory Monitoring: Verify what changes in the laws and regulations concerning the data are occurring.
For instance, an MNC has to follow the GDPR of Europe and the CCPA regulations of California if the data being processed is transferred from one area to another.
8. Data Analytics and Insights
Analytics is not generally considered one of the four pillars of data governance; however, it is a critical component of this phrase. Governance influences the quality of analytical outcomes by providing the possibility to trust data and have access to it.
Key aspects include:
- Data Preparation: Refine raw data to make usable data to the organization or to any individual in need of data.
- Visualization Tools: Dashboards are useful when it comes to communicating insights.
- AI and ML Integration: Utilize artificial intelligence to make decisions concerning governed data.
For instance, a marketing team shall apply governed customer data to come up with campaigns that will enhance engagement and, therefore, increase the returns.
Data Governance Best Practices
1. Secure Executive Sponsorship
Various studies show that the implementation of data governance initiatives cannot work well if top management does not support it. Managers are responsible for giving out the financial and other support, and the permission, as well as drawing attention to the requirements of governance policies for different divisions.
Key actions include:
- The development of a business argument is based on the necessity and benefits of data governance (less risk, improved insights).
- Organizing the project to incorporate executive project management reference to steering committees.
- Illustrating planning, decision making, and execution with quick wins to sustain excitement and support.
For instance, an executive sponsor can include the ability to resolve disputes between departments over ownership or resources.
2. Improve Organisational Collaboration
Data governance is not the work of separate IT or data sections but the work of every department that processes data. Foster a collaborative culture by:
- Organizing seminars where working employees are enlightened about data governance is essential.
- Seeking feedback from business users to know how efficient or effective the policies are in the business.
- Introducing cross-functional focused work teams which include members from IT, legal, operation, and any other functional departments.
For example, in discussing how it will be that data will be classified, particular teams such as the marketing teams will be brought on board to ensure that their specific needs are considered so that they do not have to wait for a long time to execute their campaigns.
3. Start Small, Scale Gradually
Data governance isn’t a sprint, it’s a marathon. This kind of approach is a good way to start because it enables the early trials of the strategies and discovering those chinks in sneak-up.
- Pilot Programs: Start with an example application, for instance, better data quality on the customer profile.
- Iterative Improvements: Not all initiatives should start from scratch, and you should take lessons from the pilot programs and adjust your method.
- Scalable Frameworks: Make policies and tools that one can have as a growing organization.
For instance, a company might first adopt an effective governance of its sale-related information and then it can expand into other areas such as human resource and financial data information governance programs.
4. Leverage the Right Tools
Information technology is a key driver of data governance. It means that the appropriate instruments can help to control and monitor operations, introduce restrictions, and reveal tendencies in the received information.
Key capabilities to look for include:
- Data Catalogs: These can be used for filing, cataloging, and labeling datasets.
- Data Quality Tools: For purposes of monitoring and data cleaning.
- Access Management Solutions: In surmounting and monitoring who views what information.
- Metadata Management Platforms: To make data well documented so that it could be easily discovered whenever required.
For instance, a financial sector may employ an AI assistant to alert the sector in the event of irregularities noticed in the transactions in the firm to adhere to the AML policies.
5. Develop Comprehensive Policies
Policies are part of data management strategies, or rather, how information is managed throughout its life cycle. Key areas to cover include:
- Data Classification: Create subtypes of messages (e.g., public, confidential, restricted) and appropriate action with them.
- Data Retention: Explain what retention period applies to distinct forms of data.
- Access Control: Set permission on who can see the data and who can edit or share the data.
Make sure that policies are properly written, are latest, and disseminated to all staff.
6. Communicate Successes
Reminding stakeholders of the advantages and achievements of data governance can ensure that there is continued backing and support. Share metrics, case studies, and testimonials to demonstrate:
- What risks have been eliminated or what compliance has been enhanced by governance?
- Cost reductions resulting from enhanced data access and control.
- Benefits that corporations can obtain from high quality and available data for business use.
For instance, for proving that data governance was instrumental in raising overall sales by 20% because of improved customer understanding, people get motivated to continue investing in such an effort.
Conclusion
Data governance is not an activity that can be implemented once and forgotten; it is a process. With amplified data volumes being captured by different organizations, it becomes necessary to have strong frameworks for managing such information, not just for the improvement of the organization’s performance, but also to operate within legal requirements and minimize the impacts of security risks.
By being more strategically thoughtful about where and how the organization applies data governance, your organization can unlock new value and act as a catalyst for innovation, growth and trust in an increasingly complex world.